Limitations of Current Biomedical Text Mining Community Challenges
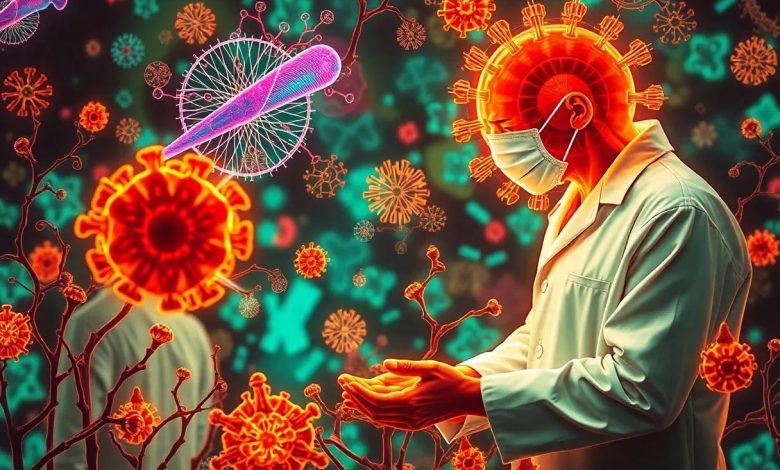
Ties
Summary and 1. Introduction
2. Overview of community challenges and 2.1 CCK
2.2 Chip and 2.3 CCIR, CSMI, CCL and DCIC
3. Presentation of evaluation tasks and 3.1 information extraction
3.2 Classification of the text and similarity of the text
3.3 Knowledge graphic and questions answer
3.4 Text and knowledge generation reasoning and 3.5 language model assessment
4. Translational computer in the extraction of biomedical text
5. Discussion and perspective
5.1. Contributions from community challenges
5.2. Limits of current community challenges
5.3. Future perspectives in the era of large -language models and references
Legends and paintings of figures
There are certain limits in current tasks for assessing community challenges on the exploration of biomedical text.
First, there is a lack of representativeness of data in evaluation tasks. Biomedical and health data have problems of sensitivity and confidentiality, which makes it difficult to obtain large -scale datasets. This results in many tasks using small or synthetic data sets, which can limit representativeness and applicability. Data quality and annotation are also difficult, as the annotation of medical data is complex and requires high levels of expertise in annotators. For data types, many tasks collect only one data type. In the future, the task organizer should consider incorporating data from several sources and methods. In addition, some data sets are limited for use only during evaluations and not made available thereafter, which limits the impact of tasks for assessing community challenges.
Second, the solutions developed can lack sufficient innovation and have poor reproducibility. Some participants tend to apply established methods to obtain quick results, rather than exploring new and innovative approaches. This can lead to a lack of innovation and diversity in methods, which limits additional technical progress. On the other hand, some algorithms can work well on specific data sets but fail to generalize to others, by reducing their large applicability and their reliability. To encourage innovation, future community challenges should highlight the exploration of new techniques and reward participants for their creativity. During integration with existing systems, the performance of algorithms must be assessed on several data sets and scenarios to ensure robustness and generalization.
Finally, there is a gap between evaluation tasks and applications in clinical practice. These tasks are generally abstract with complex and simplified world problems to facilitate evaluation and comparison. However, such simplification may not reflect the complexity, diversity and ambiguity of problems in clinical contexts in the real world. For example, several tasks focus on fundamental NLP tasks, such as extraction of medical information and classification of the text, while more complex, closely aligned on clinical practice are lacking. In clinical practice of the real world, models must always manage more noise and uncertainty, which may not have been fully taken into account in current evaluation tasks. Therefore, even systems that work well in evaluation tasks may not be able to obtain similar results in practical applications.
Authors:
(1) Hui zong, Joint Laboratory of Artificial Intelligence for Critical Care Medicine, Department of Critical Care Medicine and Institutes for Systems Genetics, Frontiers Science Center for Disease Related Molecular Network, West China Hospital, Sichuan University, Chengdu, 610041, China and the author contributed equally;
(2) Rongrong wu, Joint Laboratory of Artificial Intelligence for Critical Care Medicine, Department of Critical Care Medicine and Institutes for Systems Genetics, Frontiers Science Center for Disease Related Molecular Network, West China Hospital, Sichuan University, Chengdu, 610041, China and the author also contributed;
(3) Jiaxue Cha, Shanghai Key Laboratory of Signaling and Disease Research, Laboratory of Receptor based on receptors, Center for Collaborative Innovation for Brain Sciences, Sciences and Technologies of the Life School, Tongji University, Shanghai, 200092, China;
(4) Erman Wu, Joint Laboratory of Artificial Intelligence for Critical Care Medicine, Department of Critical Care Medicine and Institutes for Systems Genetics, Frontiers Science Center for Disease Related Molecular Network, West China Hospital, Sichuan University, Chengdu, 610041, China;
(5) Jiakun Li, Joint Laboratory of Artificial Intelligence for Critical Care Medicine, Department of Critical Care Medicine and Institutes for Systems Genetics, Frontiers Science Center for Disease Related Molecular Network, West China Hospital, Sichuan University, Chengdu, 610041, China and Department of Urology, West China Hospital, Sichuan University, Chengdu, 610041, China;
(6) Liang Tao, Faculty of Commercial Information, Shanghai Business School, Shanghai, 201400, China;
(7) Zuofeng Li, Takeda Co. Ltd., Shanghai, 200040, China;
(8) Buzhou Tang, IT department, Harbin Institute of Technology, Shenzhen, 518055, China;
(9) Bairong Shen, Joint Laboratory of Artificial Intelligence for Critical Care Medicine, Department of Critical Care Medicine and Institutes for Systems Genetics, Frontiers Science Center for Disease Related Molecular Network, West China Hospital, Sichuan University, Chengdu, 610041, China and a corresponding author.