Tired of Copy-Pasting Hive Output? This PySpark Hack Fixes It
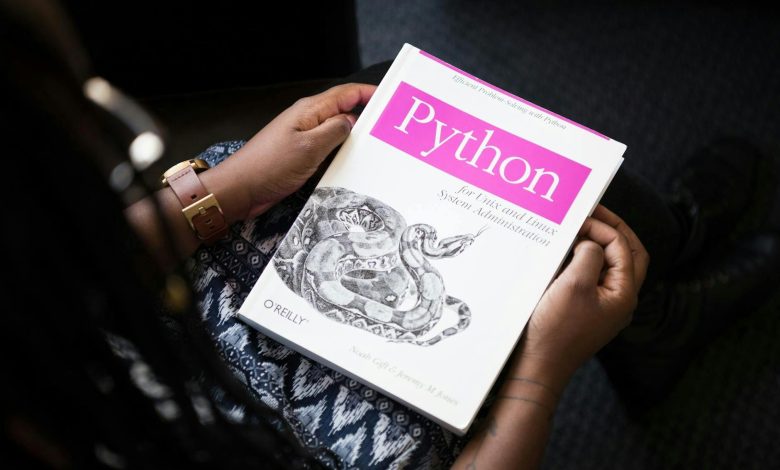
As a data engineer, I lost a lot of time furious for the simple task of hiveing the Hive or Impala console with an available CSV file.
Problem:
I have an employee table in Hive (may be impala or spark job logs), I will conduct a query on the Hive employee table that will provide console output like this:
+-----+------------------+-------+
|empid|empname |salary|
| 1| Ram Ghadiyaram| 10000|
+-----+-------+----------+--------+
I would like a quick export of CSV for the upper result of the concluded console query.
Why 🙃:
One of the many usecases is in my unit test cases, I need to use this CSV file with significant data from any database or spark job log (for example in organizing a production issue with production data from an email from the reliablity engineer ie sre's reliablity engineer site) to copy data or schema issues.
To the highly confidential world such as FinTech banks, insurance companies .. It is not possible to login in labor and see data from Hive or Impala or Spark Job Log with data etc … 😅
Below is the Pyspark method to achieve CSV from the output of the console (can be tried directly from Intellij). Here I use '|'
As a delimiter to parse from the spark directly and also exclude the schema. So this data set is exactly the console output replica.
import os
import re
import sys
from pyspark.sql import SparkSession
os.environ['PYSPARK_PYTHON'] = sys.executable
os.environ['PYSPARK_DRIVER_PYTHON'] = sys.executable
spark = SparkSession.builder \
.appName("String to CSV") \
.getOrCreate()
# Input data as a string
input_data = """
+-----+------------------+-------+
|empid|empname |salary|
| 1| Ram Ghadiyaram| 10000|
+-----+-------+----------+--------+
""".replace("|\n","\n").replace("\n|","\n")
#remove +-----+-------+------+ from the string
input_data = re.sub(r'\n[+-]+\n' , '\n', input_data)
# Capture the input data as a string
df = spark.read.option("header","true").option("inferSchema","true").option("delimiter", "|").csv(spark.sparkContext.parallelize(input_data.split("\n")))
df.printSchema()
df.show()
# Specify the path where you want to save the CSV file
output_path = "./output1.csv"
# Write the DataFrame as CSV
df.coalesce(1).write.csv(output_path, header=True)
# Stop the Spark session
spark.stop()
Cleaning input:
Combines all steps together:
Before :
+-----+------------------+-------+\n
|empid|empname |salary|\n
| 1| Ram Ghadiyaram| 10000|\n
+-----+-------+----------+--------+\n
Then : Border lines are lost, leaving rows of header and data with internal pipes. Extremely newlines at the beginning and end is harmless, such as input_data.split("\n")
And the CSV parser will ignore the empty lines.
\n
empid|empname |salary\n
1| Ram Ghadiyaram| 10000\n
\n
Now Converts to CSV using the bottom code
df = spark.read.option("header","true").option("inferSchema","true").option("delimiter", "|").csv(spark.sparkContext.parallelize(input_data.split("\n")))
df.printSchema()
# Show the result CSV data
df.show()
# Specify the path where you want to save the CSV file
output_path = "./output1.csv"
# Write the DataFrame as CSV
df.coalesce(1).write.csv(output_path, header=True)
Final output 😀
Formed CSV:
Note: For a large CSV you can compress Example:
df.write.mode("overwrite").option("compression", "gzip").csv
Why is it important:
- Unit testing: Example generate test data from queries like production (from any database/warehouse or spark log).
- Data exports: Share query results with non -technical stakeholders such as business or non -technical users.
- Pag -Debug: Get and study the output of the console during development.
- Usually parsing: SUITE WITH ANY OUTPUT OF CONSOLE OF TABLE, LIKE SPARK
DataFrame show()
Using this method of converting to CSV from any console output, I was able to copy data problems quickly for many datasets … for the descriptive purpose that demoed small data. Happy Study 😃 Happy problem solving 😀