Driving Real-Time Fraud Detection with Generative AI: Insights from Pallav Kumar Kaulwar’s Research
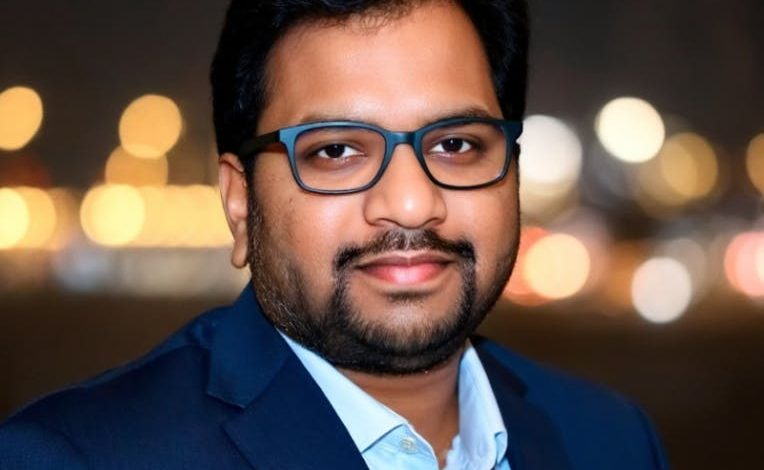
While financial systems around the world become more digitized and interconnected, the complexity and the scale of financial fraud have also reached unprecedented levels. With the advent of new technologies, cybercriminals have increased their game to circumvent conventional fraud detection systems. In response to this global crisis, the IA expert, Pallav Kumar Kaulwar, published his insightful research highlighting the role of
His article published “AI generative in financial intelligence: to unravel his potential in the assessment and compliance of risks” explains how emerging financial threats can be simulated, detected and neutralized by in -depth learning architectures such as GAN (generative adversarial networks), Bayésian neural networks and automobile variation encoders.
A new approach to fraud detection
Each year, financial frauds explain the loss of billions of dollars worldwide. Unfortunately, conventional detection methods have failed to follow this growing concern. The engines based on rules based on fixed thresholds and signatures of historical fraud are not up to technologically clever technologically cybercriminals.
In his research, Kaulwar has identified several limits of traditional fraud detection models. Most importantly, static rules can be done effortlessly by fraudsters who constantly evolve their methods with each violation. In addition, although supervised learning models are powerful, they need labeled labeled data sets, which are difficult to find for new types of fraud. Finally, traditional models are known to generate a high volume of false positives.
“The industry needs a passage from the reaction to the prediction,” explains Kaulwar. “Today's fraud detection must be dynamic, rich in data and capable of identifying new models as they emerge before they degenerate into large -scale violations.”
Kaulwar believes that the generative capacity of AI to create, simulate and test a wide range of scenarios can change the situation in this context. Institutions can proactively identify fraudulent transactions and intervene in real time by forming models to imitate normal and abnormal behaviors.
Improve financial monitoring with generative AI
Unlike traditional analysis, generating AI has the ability to generate synthetic data reflecting the financial behavior of the real world. Consequently, organizations can build robust detection models, even if they do not have in -depth fraud data games. Financial institutions can take advantage of tools such as Gans to simulate thousands of behavioral scenarios, identity models and transaction paths. Consequently, it is possible to detect vulnerabilities that are missed by static models.
Pulling this approach, Kaulwar's framework creates
In the context of LMA (fight against money laundering), complex superposition and structuring techniques used by criminals can be simulated by generative models. When used to train detection engines, these simulations can improve their sensitivity to subtle whitening signals. Generative models can also prevent payment fraud by creating a behavioral biometrics capable of identifying account controls according to the session anomalies or the dynamics of the key.
It is possible to update generative AI systems in real time with new fraud data, ensuring rapid adaptation to new attack vectors. They can also be transparently integrated into existing financial intelligence platforms.
Human surveillance in the loop
One of the key aspects of Kaulwar's research is the balance between automation and human judgment. According to him, while AI is essential for scalability and speed, human surveillance remains essential for ethical alignment, governance and contextual decision -making. The design of the man in a loop (HitL) of its frame guarantees that only experts are involved in the validation and climbing of critical fraud decisions.
The growing concerns concerning the explanation of the AI are also dealt with by the framework of Kaulwar. In addition to detecting fraud, financial institutions should explain why a particular transaction has been reported. In its generative systems, the AI (xai) modules explain a clear justification for each alert by tracing the decision path of the IA model.
This two -layer approach is essential to allow regulatory resilience. Each second is important in rapidly evolving environments such as the capital market. Although the initial detection is managed by the front line AI, the compliance teams are engaged in the examination and the response to critical incidents.
Conclusion
As fraudsters are now evolving their tactics using cutting -edge technologies, financial institutions must evolve even faster to stay ahead of them. Kaulwar's research provides an exploitable framework for the construction of intelligent, proactive, explainable and resilient fraud detection systems. His ideas can help financial institutions meet the challenges of an increasingly digital, complex and high -speed financial landscape.
“Generative AI represents a major step in the future where machines learn not only to recognize forms and models, but also have their own imagination. This imagination is integrated into our processes and our contexts, capturing human potential to interpret large financial data that has not been exploited.