Research by Harish Kumar Sriram Proposes AI-Driven Automation for Secure Transactions
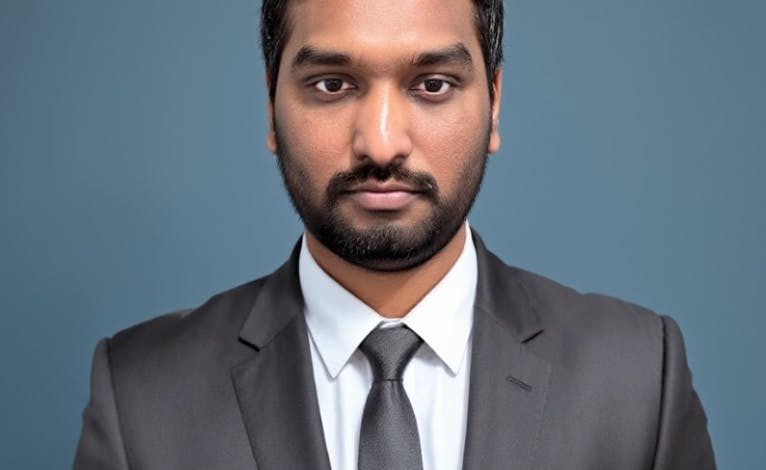
In recent years, digital financial transactions have become more common and much faster. Although it is an essential development, the landscape of threats surrounding these transactions has also become more and more complex. Identity and fraud to payments to synthetic financial crimes and data violations, guaranteeing transactions security is now an absolute priority in the world. Unfortunately, these emerging threats are often not detected when using traditional rules based on rules.
Harish Kumar Sriram, an expert deemed to process secure payments, credit risk assessment, prevention of identity theft and marketing automation, has proposed an AI -focused framework which returns the security of transactions via its research article entitled “Generative automation focused on AI in integrated payment solutions: processing of financial transactions with reactivated information to a network neural. Taking advantage of neural networks, generative AI and intelligent pseudo-labeling, its study highlights the role of automation in proactive detection of fraud, user protection through payment ecosystems and compliance.
Transactions safety in the digital age
Financial transactions occur at an unprecedented speed and evolve the current digital economy, extending on electronic commerce platforms, traditional banks, mobile applications and fintech startups. Complex safety challenges are introduced by this proliferation of digital payment interfaces. These transactions involve transit of sensitive financial data. The exploitation of even minor vulnerabilities can lead to data violations, massive financial loss and a loss of confidence in consumers.
In his research, Sriram maintains that it is time to remove inherited systems and static algorithms based on rules and kiss
By integrating AI models capable of analyzing compliance indicators and risking continuously, institutions can ensure real -time compliance with complex and scalable regulatory landscapes while reducing the load of manual and audits.
Importance of generative networks of AI and neurons
A powerful combination of neural network architectures and
Smart Pseudo-Lalmorling Framework is another important innovation presented by Sriram in his article. Using initially not marked or semi-marked data, it can form supervised AI models. Even in the categories of complex or ambiguous transactions, these models can improve their classification precision by allocating probabilistic labels to unknown data points and by refining them thanks to iterative learning. This capacity can be extremely useful for the detection of atypical behavior which indicates a risk but does not comply with known fraud models.
Sriram has used deep neural networks that can capture multidimensional relationships between data points, which are used later to generate real -time alerts or approvals. To simulate high -risk scenarios and assess system resilience against synthetic fraud, it has also incorporated generative opponents (GAN). These simulations are essential to strengthen AI capacity to operate in real environments.
Real -time fraud detection
The traditional idea of detecting fraud revolved around engines based on rules, manual audits and black lists. Although these methods are effective to a certain extent, they are often slow, reactive and incapable of managing the complexity of modern financial behavior. Sriram's research unveil an automated, intelligent and anticipated model propelled by automatic learning and analytics of data in real time.
Sriram's fraud detection frame uses a hybrid system that combines neural networks, detection of real -time anomalies and blurred logic. By monitoring continuous transaction flows, this system identifies known fraud models as well as emerging anomalies which are often missed by traditional systems.
One of the most important aspects of this system is its capacity for contextual analysis. Instead of carrying out an isolated evaluation of transactions, he analyzes behavior groups in the categories of expenditure, time zones, devices and historical trends. This allows the system to differentiate real fraud and legitimate but unusual activity.
The document also explains how models can be formed on synthetic attack vectors by simulating fraudulent transactions using GAN. By learning to recognize behaviors such as unauthorized cross -border activity, location jump, transactions division and identity masking, models become very effective in protecting institutions as well as individual users.
Final reflections
Harish Kumar Sriram's research provides futuristic vision for intelligent and secure financial transactions fed by a generative AI. With an in -depth emphasis on real -time fraud prevention, automation compatible with the neural network and ethical AI practices, this initiative has the potential to define a new reference for innovation in payment technology.
“The generative AI offers capacities to simulate, predict and optimize large-scale transaction processes, while preserving security and compliance,” he said. “Our goal is to build payment ecosystems that are self-learning, resilients to fraud and capable of adapting in real time to the change in financial behavior.”