LLM Probabilities, Training Size, and Perturbation Thresholds in Entity Recognition
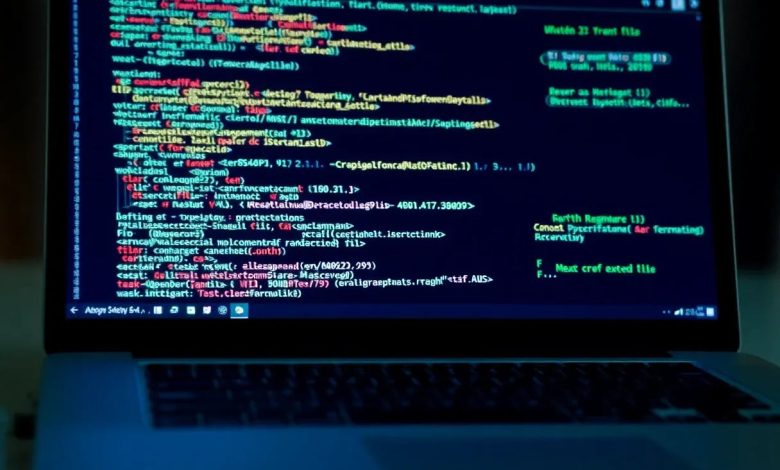
Those with -set:
.[email protected]);
(2) Pierre Lison, Norwegian Computing Center, Gaustalleen 23A, 0373 Oslo, Norway;
(3) Mark Anderson, Norwegian Computing Center, Gaustalleen 23A, 0373 Oslo, Norway;
.
.
Link
Abstract and 1 Introduction
2 background
2.1 definitions
2.2 approach to NLP
2.3 Publishing privacy data
2.4 Differences in Difference -It
3 datasets and 3.1 text anonymization benchmark (tab)
3.2 Wikipedia biography
4 Privacy-Oriented Entity Recognition
4.1 Wikidata Characteristics
4.2 Silver Corpus and Model Fine-Tuning
4.3 Analysis
4.4 Label Disagreement
4.5 misc semanty type
5 Privacy Danger indicators
5.1 LLM probabilities
5.2 SPAN CLASS
5.3 perturbations
5.4 Sequence Labeling and 5.5 Web Search
6 Analysis of Privacy Danger indicators and 6.1 Evaluation metrics
6.2 Results of Experimental and 6.3 Discussion
6.4 combination of risk indicators
7 conclusions and work in the future
Expression
References
Appendices
A. Person owners from Wikidata
B. Parameters of Entity Recognition Parameters
C. Label Agreement
D. LLM probabilities: Base models
E. Size of training and performance
F. Perturbation thresholds
A person's owner from the wikidata
The two tables below show the selected wikidata properties mentioned in Section 4.1 that make up the DEM and MISC Gazetteers.
Dem -related attributes
Characteristics with related misc
B Entity Recognition Parameters
C label agreement
Frequently confused label pairs (see Section 4.4) are shown in Fig. 4.
D LLM Probability: Base Models
Table 11 describes the (ordered) models based on the autogluon tabular predict
E training size and performance
Figure 5 shows the F1 mark of both the tabular and the Multimodal Autogluon predictor (LLM probability Section 6.3 and SPAN Classification Section 6.3 respectively) in different training sizes for both datasets. We use a random sample of 1% to 100% for each split dataset split.
F perturbation thresholds
Figure 6 shows the performance of different perturbation thresholds for both datasets for split dataset split, along with a black line indicating the threshold used in section 5.3 for review.