Integration of the bearing and research data: methodology for activity and motivation research
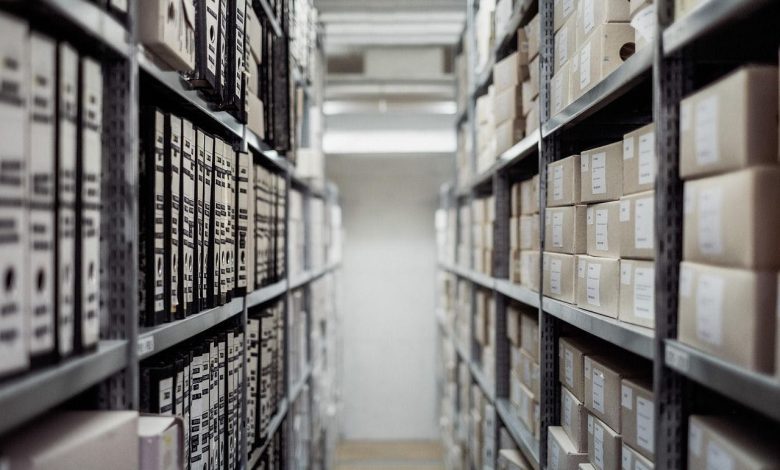
Authors:
(1) Muhammad Zia Hydari, Katzi Business School at Pittsburgh University and corresponding author;
(2) Idris Adjers, Pamplin Business College;
(3) Aaron D. Striegel, Department of Computer Science and Technology at Notre Dame University.
Table
Summary and 1 Introduction
2. Background and 2.1. Charts
3. Impact of healthy physical activity and 3.1. Competition
3.2. Social influence
3.3. Moderating impact of pre -activity levels and the size of the leaderboard
4. Data and model
4.1. Data
4.2. Model
5. Estimation and durability of the main effects of the preliminary plates
5.2. A drinking check to start the leaderboard
5.3. Fitbit's compliance
5.4. Fitbit Friction, De-Adoption of the Ranking and Additional Durability Control
6. Heterogeneous influence of the leaderboard
6.1. Heterogeneity according to the level of prior activity
6.2. The interaction of the size, rank and prior activity of the leaderboard
6.3. Summary of Heterogenic Effect analysis conclusions
7. Conclusions and discussion, end notes and references
4. Data and model
4.1. Data
We use a unique panel data set, consisting of 516 bachelor's studies at the US University from October 2015 to September 2017.[11] This set of data consists of data and periodic research data for granulated equipment. For the data of the device, students were offered Fitbit Charge HR devices, which were then used to record their physical activity. We will access three types of Fitbit data: (i) the number of steps that are daily access; (ii) Ranking data that reflect if the focus student has a leaderboard and, if so, a seven-day average step by step to determine the ranking of the participants' leaderboard; and (III) by minute heart rate data. Students synchronize their data with the Fitbit platform either through the Dongl and Desktop App or Smartphone Application. We implemented a customer application that invited Fitbit Apps (API) to download data from synchronized students to download data to download data to download data. The customer application was a set of scripts that worked automatically every night. All participants in the study explicitly provided our customer application to allow access to their data through Fitbit APIs.
Step measurements take place only if students carry their fitbit devices regularly. We use the term compliance to refer to the regularity with which students carry their fitbit device. We calculate following the heart rate data, assuming that the student wears his / her fitbit during the day when the pulse reported is zero. Students were paid $ 20 for maintaining at least $ 40% and regular sync with Fitbit servers. Fitbit Charge Mr. could save up to seven days of data on the spot, so synchronization beyond the seven -day interval would cause lost data and lower compliance.
Fitbit Check Mr. Measurements We use as a result of this study are quite accurate. Validation studies in laboratory and natural environments have found that the average absolute percentage of Fitbit Charge Mr. is less than 10%, except for very mild activity (Wahl et al. 2017, Bai et al. 2018). Bai and others. (2018) also found that Fitbit Charge HR heart rate measure was a mape ≈ 10%, although other studies have found mixed results. Even if the heart rate of heart rate was higher, our study is unlikely to be negatively affected. We use the pulse only to measure compliance, so that any zero value is interpreted as a device that the participant uses at that moment.
Participants were also asked to complete an intense study at the beginning of the study and were asked to do shorter surveys with six -month waves to refresh the key measures. These studies significantly provided data on demographic data (gender, religious affiliation, parents' income, etc.), psychological attributes using validated scales (personality, self -regulation), social communication and ability (trust, anxiety, etc.), technology (use of social media, mobile applications, etc.) and health status (body mass, etc.). Although most students took a study, there was no response because these studies were not mandatory. On average, students completed three waves of research data (about six months apart). We use this data in two ways. We mainly use the appropriate research data to model the tendency to select in the leaderboard and, together with advanced weighing methods, construct a weighted sample that achieves the covarate balance between the introductions and the non -deployment of the charts. Second, we use a subset of research data to generate controls, which include individuals changing time -specific features that may be related to both the introduction of the leaderboard and the physical activity, and to check the durability of our main results.12 Table 1 presents descriptive statistics outcome, treatment and some demographic variables.