How do Privacy Risk Affect Text Disinfection
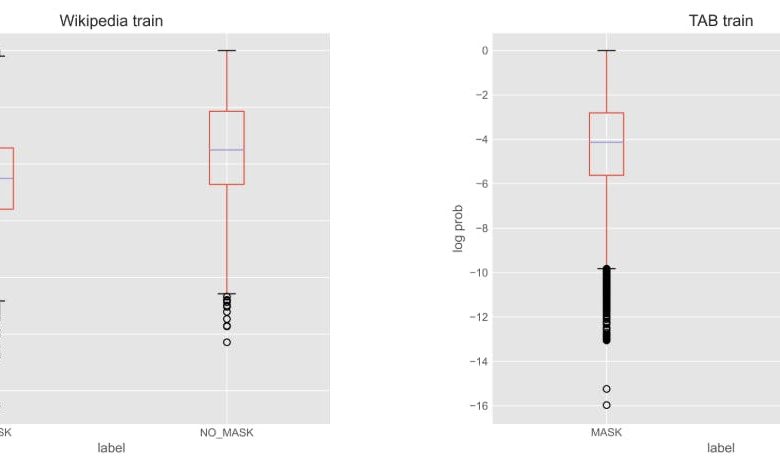
Authors:
(1) Anthi Papadopoulou, Language Technology Group, Oslo University, Gaudadalleen 23b, 0373 Oslo, Norway and corresponding author ([email protected]);
(2) Pierre Lison, Norwegian Calculation Center, Gaudadalleen 23a, 0373 Oslo, Norway;
(3) Mark Anderson, Norwegian computing center, Gaudadalleen 23a, 0373 Oslo, Norway;
(4) Lilja Øvrelid, Language Technology Group, Oslo University, Gaudadalleen 23b, 0373 Oslo, Norway;
(5) Ildiko Pilan, Language Technology Group, Oslo University, Gaudadalleen 23b, 0373 Oslo, Norway.
Table
Summary and 1 Introduction
2
2.1 Definitions
2.2 NLP approaches
2.3 Disclosure of Privacy Maintain Data
2.4 Difference Privacy
3 Data sets and 3.1 Text anonymized benchmark (tab)
3.2 Wikipedia biographies
4 Privacy -oriented item recognition
4.1 Wikida properties
4.2 Silver Corpus and Model Fine Tuning
4.3 Evaluation
4.4 Tag Disagreements
4.5 MISC Semantic Type
5 Privacy risk indicators
5.1 LLM probabilities
5.2 Classification
5.3 PERTURBATIONS
5.4 Song Signing and 5.5 Web Search
6 Analysis of Privacy Risk and 6.1 Assessment Metrics Analysis
6.2 Experimental results and 6.3 discussion
6.4 A combination of risk indicators
7 conclusions and future work
Declarations
References
Extras
A. Wikida human qualities
B. The training parameters of the entity of the unit
C. Tag contract
D. LLM probabilities: basic models
E. The size and performance of training
F. Perturbation thresholds
6.2 Experimental results
First, we appreciate the five risk of privacy, using the PII scope of both hand -labeled both Tab Corpus and Wikipedia biographies. The results are shown in Table 5. LLM probabilities and spa-class approaches were trained in the respective training kit (either Wikipedia or Tab) and the threshold of the disturbance-based method was adapted to the same way as a training kit. The results of the web search are based on the two alternative techniques presented in two sections 5.5 based on the intersection of URLs or the estimated number of hits. We also offer results obtained with a simple starting line that conceals all the lengths of the pipe.
We then appreciate the risk indicators with the PI-i-IT, which were actually identified by the recipient of the privacy-oriented unit described in Section 4, allowing us to evaluate the proposed approaches from the end of the end. The results are shown in Table 6. Note that in this setting, the starting line of the majority of the rule (which masks all the pi-pavements) causes the withdrawal grades, which are lower than 1, due to the detection errors resulting from the privacy-oriented entity.
6.3 Discussion
Now we are discussing the experimental results of each privacy risk indicator one by one.
Llm probabilities
We can notice that an automatic model consisting of a guided classification ensemble, such as decision -making trees with optimized hyperparameters of the development kit, outperform the lighter logistical regression model. In other words, the task of proposing high -risk texts seems to require a non -linear decision -making limit, considering the high -risk texts.
We also notice a significant difference between Wikipedia's biographies and Tab Corpus. It is particularly instructive to explore the significance of the decision to mask the sign -up options. While the PIU extends from the biographies of Wikipedia, a clear difference between masked and disguised chips log-malfunctions shows that this Tab Corpus does not apply[8]To.
The classifier reaches its optimal performance relatively quickly, after watching about 1 % of the total workout kit in both shells. Ablation attempts also show that the most important feature of the classification is the type of PI, as some types mask the human annotators much more often than others.
Span
The approach of the classification of the SPAP improves the classification performance compared to the classification that is trained only in terms of the probability of LLM, especially for the anonymized benchmark of the text.
As the training of this classification includes a large language model, it requires a little more training data than a classification based exclusively on LLM probabilities and PIA types. We are experimentally observing that the classification performance stabilizes about 10%of the training cases of both data sets. Based on the tests of Ablation, we also see that the outputs of the fine -tuned language model play a major role in the final prediction with the PI type.
Disturbances
Despite the theoretical advantages (for example, the ability to directly assess the extent to which the PI-range helps to predict a direct personal identifier), the disturbance-based method works poorly and does not seem to improve most of the rule of the rule. Indeed, the mixing mechanism with the cost function used to fix the log-malfunction leads to the vast majority of text lengths.
Tagging the sequence
In general, this approach seems to provide the best balance between accurate and recalling grades that can be expected on a large language model, which is fined according to manually marked data. Contrary to the SPA classification method, which weighs only the chips in the PI SPA, the approach to the sequence labeling can take into account the context of each range.
It should be borne in mind that the results of Tables 5 and 6 are obtained with a default threshold of 0.5 masking probability. Of course, this threshold can be adjusted to increase the relative importance of recall results, as is often the case when disinfecting the text to increase the cost of false negatives.
A partial match -based method, which considers the Pi -SPIT, is risky if at least one forming symbol is marked as risky, gives the best results. We also notice that the results of Wikipedia biographies are lower than the TAB results. This is likely to be due to the smaller number of biographies available for training (453 short texts), compared to the 1014 doctors of the Training Card Training Kit.
Web search
Table 5 shows a clear difference between the use of the intersection of the URL and the use of an estimated hit, for the first time there is a very low recovery score, while the latter shows a more balanced performance between accuracy and recovery. However, the crossing of the URLs provides a better explanation than the number of hits, as it may indicate the actual URL to the user to help to re-identify.
Although the use of the intersection of URLs is prevented by limiting the number of leaves, the likelihood of meaningful intersection between target and units reduces the likelihood of the web results we shrink. It is possible to try to analyze the text found in the URL to assess whether or not the target group is mentioned.
On the other hand, the number of hits is up to the limit, the better approximation of the risky units, despite the unreliability of the number of API's hits. Note, however, that the restrictions we have already mentioned in Section 5.5, which affect performance. Although the web can be considered as a very useful and detailed approach of possible background knowledge, which the attacker may be and the use for re -identification, the technical details of its use make it difficult to explain the cause of the performance.
Summary
As you might expect, trained data trained privacy risk indicators, in particular sequence marking, are the best performance when compared to high -risk expert comments. However, there are few text data with a masking decision note for many texts for disinfectants.
As for data utilityPW) is higher than the usual accuracy of all privacy risk indicators. As said Pilan et al. (2022), this weighted result is more informative than the main choir of accuracy as it takes into account the informativeness of each sign. As a result, a higher weighted accuracy score means that the superpower tends to take place on less informative chips.
6.4 A combination of risk indicators
Finally, we also appreciate the performance of privacy risk together. More specifically, we will deal with a manual pi-span high risk if it is marked as such at least one, two or three risk indicators.[9] The resulting performance is given in Table 7.
Although a combination of privacy risk indicators with ≥ 3 positive signals causes superposition, it identifies all direct identifiers and virtually all quasi identifiers, while maintaining more accuracy than most rules of the rule. This high recall is important in disinfecting the text, as the cost of ignoring a high-risk pi-span is much higher than the false positive cost. While superpowering slightly reduces the data utility (making the text less readable or for removing some useful content), the presence of false negatives means that it is possible to re -identify people in question and therefore their privacy is not fully guaranteed.
[8] At least when all the types of pi are merged. However, if we consider these log-type PIs, we will notice the difference in the log-failure of many types of logs.