AT&T Open source AI approach to customer service calls
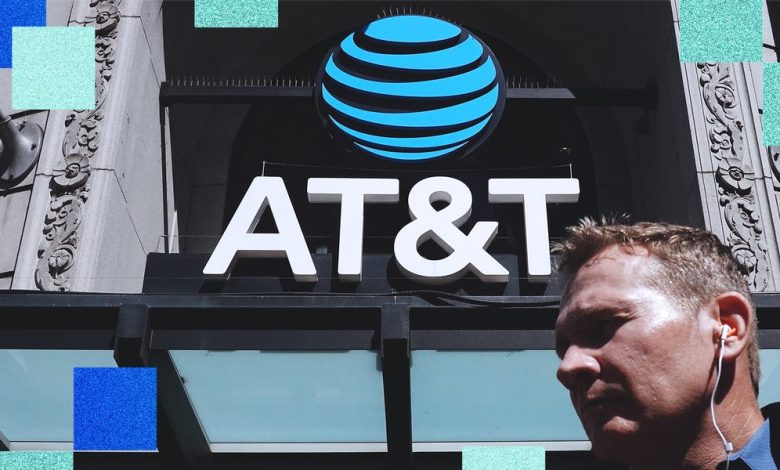
AT&T receives 40 million customer service calls a year. Some callers want to add telephone lines, register new addresses or update meetings, but many have problems with reporting. These calls contain valuable information, but it is not easy to mining.
Each of them listening to a good idea of what new problems arise and could get small problems before growing up. But if thousands of calls come every day, it would be a laborious, practically impossible task.
The transcription has been automated for some time, so AT&T made it manually. However, the employees had to read millions of summaries and put each of the 80 categories analyzed for all follow-ups that could be taken. The ultimate goal is to avoid what consumer -oriented companies call “churn”. In principle, they want to leave customers.
Hien Lam, a senior data scientist at AT&T, explained the process at the NVIDIA GTC conference in March.
Now AI can swallow and classify speeches with large language models.
Chatgpt way
It was quite simple. AT&T used Chatgpt to read and sort summary. It did a good job, but the Lam team saw the problems that came on the road.
“Although the GPT-4 model produced high quality outputs and we were able to save 50,000 customers a year, it was very expensive,” Lam said. In addition, Chatgpt customers sometimes have to wait for powerful and expensive chips to operate AI systems called graphics operating units.
Sorting calls was a daily task. “If it takes longer to run overnight, it's not a reasonable workflow,” said Ryan Chesler, an open source AI platform H2O.
So they decided to create a more flexible system that AT&T could control, but it also costs less.
Five with open source
LAM worked with Chesler, working under the theory that if they could sew several open source AI models with different “skills”, they could achieve similar results at a dramatically lower cost while keeping the company data private.
First, they distilled the GPT-4 into three smaller open source models. The most basic model was smart enough to sort about a quarter of the categories. The call, which mentioned the name of a competitor, was easy to identify. A speech about a crew member of the store demanded a more sophisticated model.
About half of the calls can be managed with an open source model called Danabe, a small but powerful model created by H2O.Ai. LAM worked with Chesler to clarify it with AT&T needs.
The most complex calls went to the Meta Llama 70B model, which is larger and more expensive. Open source models are cheaper in nature, but they are not free to start because they still need computing power. But using only larger models if necessary, the team kept the cost of the cost.
In fact, an open source solution cost 35% of what AT&TGpt cost to use, with 91% relative accuracy, LAM said. It was also faster.
“Using GPT-4, it took 15 hours to process one day's summary. It took just under five hours in our new solution,” Lam said.
Next, they want to accelerate things even further.
“Because it takes 4.5 hours for the whole day, we want to do it in real time after hanging with AT&T,” Lam said. “We could get these outputs right away.”