Analyzing User Preferences and Performance in VR Tasks
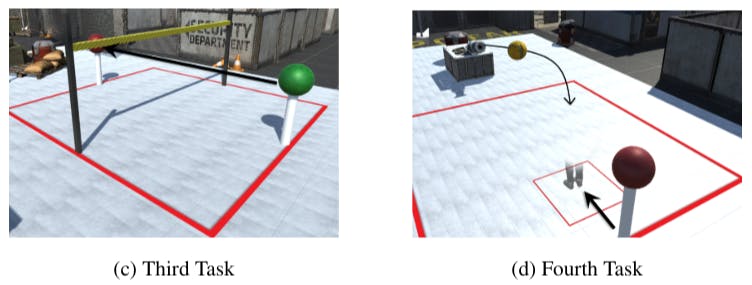
Ties
Summary and 1 Introduction
2 related work and 2.1 virtual avatars
Visualization of the cloud of 2.2 points
3 Test design and 3.1 Configuration
3.2 User representations
3.3 Methodology
3.4 Virtual environment and 3.5 tasks Description
3.6 questionnaires and 3.7 participants
4 results and discussions, and 4.1 User preferences
4.2 Task performance
4.3 Discussion
5 conclusions and references
4 results and discussion
In this section, we present the main observations made during tests as well as the difficulties and suggestions of users on
The test task. To assess the difference between the representation embodied by the three users from the first and third person perspective, we have collected objective and subjective data respectively, in the form of newspapers and requests for information during the evaluation sessions. For the continuous variable, that is to say time, we used the Shapiro-Wilk test to assess the normality of the data. Given that all the samples were normally distributed, we used repeated anova measurements with the correction of Bonferroni applied to post-hoc tests for multiple comparisons and the test test of samples paired between two samples, to find statistically significant differences. For discreet data, such as the number of obstacle or response collisions to the questionnaire, we used Friedman's non-parametric test with the post-hoc test signed by Wilcoxon, also with a bonferroni correction.
In the following sub-sections, we present the analysis carried out on the basis of the results of the questionnaires and the data of log files obtained during the test.
4.1 User preferences
We have made two different comparisons based on the data collected via the questionnaires, between representations on the same perspective and the same representations between perspectives.
4.1.1 Perspective
By comparing between representations in the first person perspective, we have found no statistical difference in the direction of the incarnation, the ease of finishing the task and the fatigue felt during the test. The only exception was on Q8, where users estimated that it was easier to perform the second task (pass under the obstacle) with the abstract representation compared to the punctual representation (Z = -2.64, p <0.05).
By comparing between representations from the third person perspective, the results were slightly better. Users have attributed a higher production mode of realization to point avatar by comparing to the mesh avatar, with statistical meaning only on the agency meaning (Z = -2.812, p = 0 <0.01), that is to say a feeling of control of the virtual body. Even if users felt more fatigue with the Avatar PointCloud against the mesh avatar (Z = -2.53, p = 0.011), on the prospect of the third person.
4.1.2 Representation
On the mesh avatars, users felt a stronger sense of the incarnation in the first person perspective in all its subcomponsors: agency sense (Z = -2.687, p = 0.007), corporal owner (z = -2.775, p = 0.006) and Auto -Lieu (Z = -3.574, p <0.005). They also found easier to walk in the virtual environment using 1PP (Z = -3.352, p = 0.001). Regarding the ease of tasks, they overall preferred the mesh avatar in the first apple, but with statistical significance only in tasks 1 (Z = -2.902, p = 0.004) and 3 (z = -3.579, p <0.005), Q6 and Q8 respectively.
The avatars at Cloud Point had a sensation of production mode similar in both perspectives, but users found easier to perform the third and fourth tasks, Q8 (Z = -2.771, p = 0.006) and Q9 (Z = -3.695, p <0.005) respectively.
The abstract representation had a stronger sense of body property and self-localization, but with statistical significance only on self-package (Z = -3.422, p = 0.001). Users have also found easier to walk the virtual environment (Z = -2.838, p = 0.005), to avoid obstacles in the first tasks (Z = -2.638, p = 0.005) and third (Z = -3.879, p <0.005) and on the damage task (Z = -2.676, p = 0.007).