AI breakthrough exacerbates telescope images astronomy next big leap
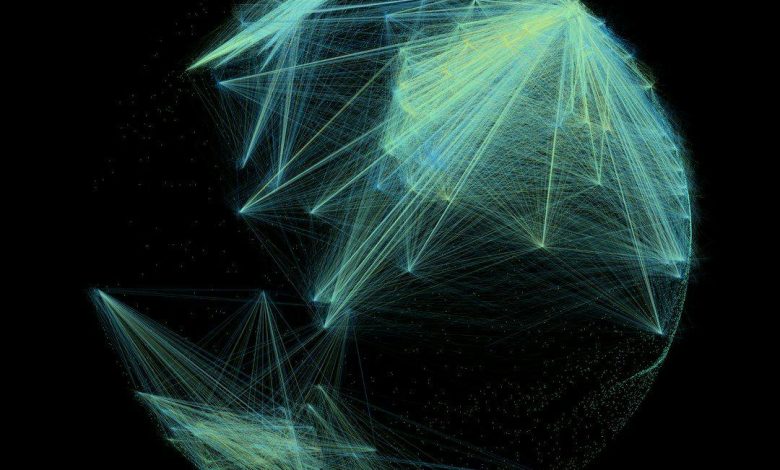
Authors:
(1) Hyosun Park, Department of Astronomy at the University of Yonsii University of Korea;
(2) Yongski Jo, an artificial intelligence school, sleep, Ulsan, Republic of Korea;
(3) Seokun Kang, School of Artificial Intelligence, Sleep, Ulsan, Republic of Korea;
(4) Taehwan Kim, a school that graduated from artificial intelligence, sleep, Ulsan, Republic of Korea;
(5) M. James Jee, Department of Astronomy at the University of Yonsii, Soul, Republic of Korea and Department of Physics and Astronomy, California University, Davis, Ca, USA.
Table
Summary and 1 Introduction
2 method
2.1. Overview and 2.2. Architecture of the Coder-Deplifier
2.3. Transformers to restore the image
2.4. Details of implementation
3 data and 3.1. HST data
3.2. Galsim data
3.3. Jwst data
4 JW test data results and 4.1. PSNR and SSIM
4.2. Visual inspection
4.3. Restoring morphological parameters
4.4. Restoring photometric parameters
5 Application for real HST pictures and 5.1. Restoring and comparison with diverse pictures of one-e-success
5.2. Restoring and comparison with multilateral jwst images
6 restrictions
6.1. The decomposition of recovery quality due to high noise level
6.2. Test for the point of the point sources
6.3. Artefacts of pixel correlation
7 conclusions and recognitions
Appendix: A. Image recovery test with empty noise pictures
References
Summary
Transformer architecture has revolutionized in the last several years in the field of deep learning in various fields, including natural language processing, code generation, recognition of images, forecasting time series, etc. We propose to implement Zamir and others for an effective transformer for deconvolution and to improve astronomical images. We conducted experiments using high quality images and their impaired versions, and our deep learning model demonstrates the extraordinary restoration of photometric, structural and morphological information. Compared to the terrestrial truth of JWS-images, the advanced versions of our HST quality images reduce the scattering of isofotal photometry, serial index and semi-light radius according to factors 4.4, 3.6 and 4.7 respectively, Pearson correlation coefficients. The performance breaks down if the input images correlate with noise, point -like sources and objects. We assume that this deep alot model turns out to be valuable for many scientific applications, including precision photometry, morphological analysis and shearing.
Keywords: Techniques: Image processing – galaxies: basic parameters – galaxies: photometry – galaxies: structure – Sõrvõpe – Astronomical Databases: Pictures
1. Introduction
The deeper and sharper image of the astronomical object offers the opportunity to gain new knowledge and understanding. One of the most outstanding examples is the advent of James Webb's Space Telescope (JWS), launched in 2021, which has been constantly expanding our knowledge of the universe through its discoveries since its discoveries due to its unprecedented resolution and depth. This trend is reminiscent of a similar era three decades ago when the Hubble Space Telescope (HST) became the most powerful tool for us at the time.
The continued efforts of astronomers to increase the depth and clarity of astronomical shooting and clarity extend beyond astrations to cover important developments in the algorithmic domain. Early techniques relied on straight Fourier deconvolution techniques (eg Simkin 1974; Jones & Wykes 1989). The main challenge of this approach is the linear filtration of the noise amplification and the frequency -dependent signal and noise ratio (eg Tikhonov & Goncharsky 1987). However, the filtering method only provided the results of the strip. The development of computer technologies introduced the calculated demanding and complex approaches based on Bayes' principle with various regulatory schemes (eg Richardson 1972; Lucy 1974; Shepp & Vardi 1982). It was shown that some applications of their Bayesian approaches are outperformed by the Early Deceasvolution methods of Fourier. However, regulation could not restore both compact and extended functions at the same time. To overcome this restriction, several ways of resolution or spatially adaptive approaches were recommended (eg Wakker & Schwarz 1988; Yan et al. 2012).
In recent years, the onset of in -depth education has significantly influenced the overall area of the recovery and improvement of the image. There is also an increasing number of studies in astronomical contexts (eg Schawinski et al. 2017; Sureeau et al. 2020; Lanse et al. 2021; Akhuryy et al. 2022; Sweeere et al. 2022). Deep learning algorithms, especially convolutionary nerve networks (CNNS; Krizhevsky et al. 2012), has shown promising results from images automatically in learning and enhancing their depth and resolution (eg Zhang et al. 2017; d´ıaz Baso et al. 2019; Elhakiem et al. 2021; Zhang et al. By training, deep learning models can learn the complex patterns and relationships of the data, allowing for a more accurate and customized image.
Deep learning techniques offer advantages with traditional methods, such as Fourier Donvolution and Bayesian approaches, providing greater flexibility and adaptability to various databases. CNN integration with other deep -profile architectures, such as generative racing networks (Gans; Goodfellow et al. 2014) and repetitive nerve networks (Rnns; Williams; Zipser 1989) have further expanded the capabilities of the image enlargement (eg Ledig et al. 2016; Schawinski et al. Generating very realistic and detailed images by pushing the borders only to traditional methods.
Transformer Architecture (Vaswani et al. 2017), which has been in various fields over the past several years, including well-known large language models, is a revolution in the field of deep learning, can be considered a potential alternative to overcome CNN-based models. However, with its original implementation structure, which consists of so -called self -control layers, it is possible to apply the transformer module to large images, as the complexity of the calculation increases with the number of four -rated pixels.
Zamir et al. (2022) developed an innovative scheme to replace the original self-monitoring block with multi-DCONV transmission (MDTA) block. The MDTA block, which implements the domain of the function rather than the domain of the pixel, ensures that the complexity with the number of pixels only increases linearly, making this app on large images. Zamir et al. (2022) showed that their model, called Restormer, achieved a modern result to demonstrate the image, the movement of one image, the deficiency deflecting and the image denoity. However, its performance has not been evaluated in the astronomical context.
In this article, we propose to implement Zamir and others to carry out an effective transformer deconvolution and to make denosation to improve astronomical images. Specifically, we will study the feasibility of improving the HST images to achieve the quality of JW in both the resolution and the depth. We are building our model Zamir et al. (2022) Implementation and implementation and use a transmission learning approach using the model initially simplified galaxy images, and then finted with realistic galaxy images.
Our paper is built as follows. §S describes the details of the applications used in the Restormer General Architecture and the current galaxy recovery model. §3 explains how we produce training data. The results are submitted in §4. We show the results when the model is applied to the real HST images in the § and we discuss the restrictions of §6 before we end in §.