Impact of community challenges on biomedicine text mining studies
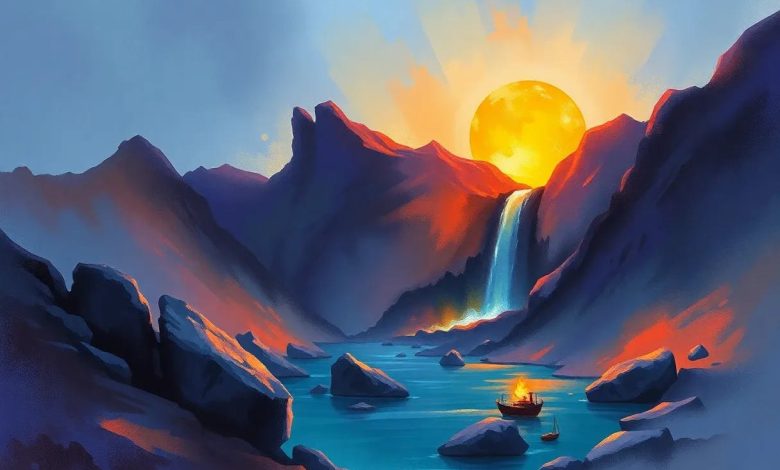
Table
2. Overview of community challenges and 2.1 CKK
2.2 Chip and 2.3 CCIR, CSM, CCL and DCIC
3. Overview of evaluation tasks and 3.1 Taking up information
3.2 Classification of the text and the similarity of the text
3.3 Knowledge graph and answer questions
3.4 Text generating and justifying knowledge and evaluating 3.5 large language model
4. Translative Informatics in the mining of biomedicine text
5. Discussion and perspective
5.1. Contribution of community challenges
5.2. Restrictions on the current community challenges
5.3. The future perspectives and references of the era of large language models
Community challenges have made a significant contribution to studies of biomedical texts in many aspects. We summarize five significant contributions, from the level of research to the level of applications, including the development of data standards and evaluation bonds, the promotion of technological innovation and development, interdisciplinary cooperation and communication, promotion of education and training,
Data sets and comparative ones
Community challenges generally release data sets related to specific NLP tasks. These data sets are manually comments on domain experts and come from many biomedical materials, such as published literature, clinical data and internet forums. These data sets are valuable resources for training and evaluating models, as well as comparative ones to compare the performance of different approaches. For example, scientists have developed a comparative cblue [64]which combines the data sets of several shared tasks and aims to assess the ability of the model to understand the Chinese biomedical language. Moreover, recently, scientists have changed numerous tasks for a quick language generation and constructed the benchmark of the assessment. [59]aimed at assessing the possibilities of large language models in the field of health.
Technology innovation and development
Community challenges encourage participants to propose top -level algorithms and methodologies to deal with the mining problems of biomedical text. This environment stimulates the innovative thinking and technological progress of scientists by leading the development and breakthroughs in the field. Taking an information out of the task as an example. Initially, early solutions were based on rule -based and dictionary -based methods. Thereafter, deep neural network methods, especially long-term memory (LSTM), achieved architecture, gradually traction and received significant attention. The participants then often used their own powerful text to be known and found applications in various biomedical fields [68]To. Recent studies also contain major language models such as Chatgpt, such as goals such as data enhancement or zero-shot mining [69]Introducing their potential even further to move forward.
Interdisciplinary cooperation and communication
Numerous experts with different research backgrounds participate in these community challenges. Those experts from academic academic and industries come together through academic conferences to show their algorithm architectures, performance results, and explore possible cooperation opportunities. In addition, the challenges of the community also offer advantages to participants through the publication of specific tasks. This interdisciplinary cooperation and communication plays between different fields in promoting knowledge sharing and cooperation, thereby motivating the development of biomedical text mining.
Education and training
Evaluation tasks are valuable for students and beginners of the Bionl Domain as learning and practical resources. They allow participants to focus on specific tasks, participate in competition, discuss with experts and improve their skills by evaluating their methods and receiving constructive feedback. After completing the task, the researchers can further use the data set to evaluate the algorithms and publish the articles. In addition, these shared tasks also provide open access to relevant data sets that universities often use for teaching courses and practical exercises to assess students' learning outcomes. It is worth noting that some data sets have explicitly stated to use their restriction only during the community challenges and are not made available after that.
Translative Informatics application
Mining biomedical text can significantly contribute to the standardization of knowledge. By taking out a large amount of valuable information on the large amount of biomedical literature, it is possible to create high -quality basics and ontologies. These resources, in turn, can provide scientists, doctors and policy makers timely evidence -based information. For example, the mining of semantic relationships from genes from literature can help scientists discover novel gene candidates for disease [70]While mining Picos information from literature can contribute to the bases for clinical evidence knowledge [71]To. The mining of biomedical text plays a crucial role in supporting clinical decisions. Through the extraction and normalization of clinical information, large quantities of non -structured clinical text data can be transformed into structured and calculated. This transformation allows doctors to access the comprehensive and accurate patient information, facilitating clinical diagnosis and treatment recommendations. In addition, the techniques of mining biomedical texts can also be used to predict and risk evaluation of epidemic trends. By mining extensive clinical text data, it is possible to identify the main features and patterns associated with patients, which allow appropriate intervention measures to be implemented to improve patients' health indicators. The mining of biomedical text has significant potential for commercial applications. In the challenges of the community, the company organizes repeated evaluation tasks. These tasks usually use data from real world health sources and have clear clinical application scenarios, such as patients' health consultancy and responding to automated questions. Successful development of these task solutions can provide companies with opportunities and competitive advantages.
Authors:
(1) Hui Zong, a joint laboratory for critical assistance in medical artificial intelligence, critical assistance at the Medical Department and Systems Genetics Institutes, Frontiers Science Center for Molecular Networks, Western -China Hospital, Sichuan University, Chengdu, 610041, China and author contributed equally;
(2) Rongong Wu, a joint laboratory for critical assistance in medical artificial intelligence, critical assistance at the Medical Department and Systems Genetics Institutes, Frontiers Science Center in Moleclamic Network, West -China Hospital, Sichuan University, Chengdu, 610041, China and author contributed equally;
(3) Jiaxue Cha, Shanghai Signal Laboratory, Receptor -based Biomedicin Laboratory, Brain Science Cooperation Innovation Center, Life Sciences and Technology, Tongji University, Shanghai, 200092, China;
(4) Erman Wu, a common laboratory for critical assistance in medical artificial intelligence, critical assistance at the medical department and system institutes of genetics, Frontiers science molecular mesh, West -China Hospital, Sichuan University, Chengdu, 610041, China;
(5) Jiakun Li, a common laboratory for critical assistance in medical artificial intelligence, critical assistance in the medical department and the Institutes of Genetics of Systems, Frontiers Science Center Molecular Network, West China Hospital, Sichuan University, Chengdu, 610041, China and Urology Department, West Hospital 610041, China;
(6) Liang Tao, Shanghai Business School, Shanghai, 201400, China;
(7) Zuofeng Li, Takeda Co. Ltd., Shanghai, 200040, China;
(8) Buzhou Tang, Computer Science Department of the Harbin Institute of Technology, Shenzhen, 518055, China;
(9) Bairong Shen, a joint laboratory for critical assistance in medical artificial intelligence, critical assistance at the Medical Department and Systems Genetics Institutes, Frontiers Science Center for Disease Molecular Network, West -China Hospital, Sichuan University, Chengdu, 610041, China and Author.