Concluding Remarks: Blockchain and Cryptography Convergence Insights
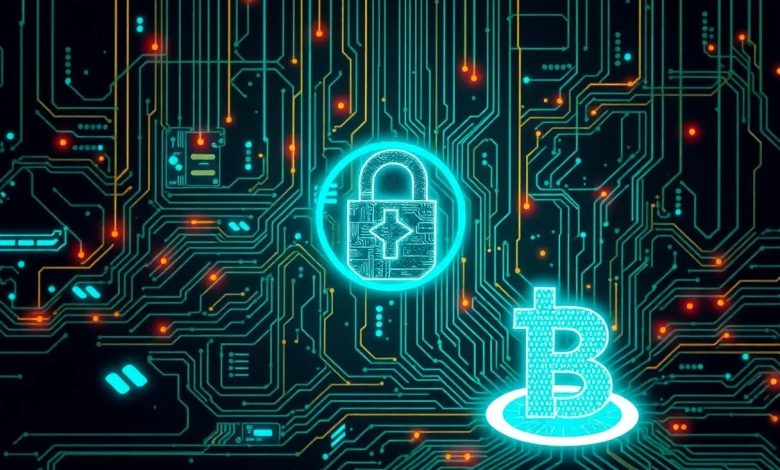
Ties
Summary and 1. Introduction
2 related works and 2.1 technological convergence approaches
2.2 Technological convergence measures
2.3 Technological convergence models
3 data
4 Method and 4.1 Proximity indices
4.2 Interpolation and adjustment data
4.3 Clustering
4.4 Forecasts
5 results and discussions and 5.1 overall results
5.2 Case study
5.3 Limits and future work
6 Conclusion and references
Appendix
6 Conclusion
In this study, we introduce a method that uses text extraction and bibliometry techniques, using the “OpenAlex” catalog, to create and predict technological proximity indices specific to encryption technologies. A case study applying our method highlights the convergence between blockchain and public key cryptography. Ideas from our method can provide precious advice to stakeholders and amateurs sailing on the impacts of encryption technology.
We recognize certain constraints in our study. Our corpus of literature was limited to “OpenAlex”, which may not in depth represent the fundamental interests and activities of the research community working on encryption technologies. In addition, the non -standardized nature of our indices obstructs direct comparisons between various data sources. These limits also suggest potential ways for future research to extend our results, enriching the field of scientific surveillance within the encryption technology community.
Additional information. The code of this project is located on https://github.com/technometrics-nab/proximity-indices-applied-To-openalelex.
Thanks. We thank Jacques Roitel, whose work was the original basis of this article. In addition, we would like to thank the other trainees working in Armasuisse when this research was carried out for their useful coding advice: the Meynent, Marc Egli, Francesco Intoci, Lucas Crijns and Alexander Glavackij.
References
[1] Zhou, Y., Dong, F., Kong, D., Liu, Y.: Depliation of the process of convergence of scientific knowledge for the early identification of emerging technologies. Technological forecast and social change 144, 205-220 (2019). https: //doi.org/10.1016/j.techfore.2019.03.014. Accessed in 2022-09-30 [2] Wang, Z., Porter, Al, Wang, X., Carley, S.: An approach to identify the emerging subjects of technological convergence: a case study for 3D printing. Technological forecast and social change 147, 723–732 (2019). Consulted 2022-11-24 [3] Carley, SF, Newman, NC, Porter, Al, Garner, JG: an indicator of technical emergence. ScienceTometrics 115 (1), 35–49 (2018). Org / 10.1007 / S11192-018-2654-5. Accessed in 2022-09-30 [4] Halaweh, M.: Emerging technology: what is it. Journal of Technology Management & Innovation 8 (3), 108–115 (2013). Org / 10.4067 / S0718-27242013000400010. Publisher: Universidad Alberto Hurtado. OPTADD OF ECONOMICIEC Y NEGOCIOS. Accessed in 2022-09-12 [5] Porter, Al, Garner, J., Carley, SF, Newman, NC: Emergence Scoring to identify border R&D subjects and key players. Technological forecast and social change 146, 628–643 (2019). Org / 10.1016 / d. TechFore.2018.04.016. Accessed in 2022-09-13 [6] Curran, C.-S., Bring, S., Leker, J.: Anticipation of convergent industries using data accessible to the public. Technological forecast and social change 77 (3), 385–395 (2010). Org / 10.1016 / J.TECHFORE.2009. 10.002. Accessed 2022-10-21 [7] Song, CH, Elvers, D., Leker, J.: Anticipation of converging technological fields – a refined approach for the identification of areas of attractive innovation. Technological forecast and social change 116, 98-115 (2017). Org / 10.1016 / J.TECHFORE.2016.11.001. Accessed in 2022-09-30 [8] Huang, L., Cao, X., Cai, Y., Ren, H., Xing, T., Ye, P. [9] Song, K., Kim, KS, Lee, s.: Discover new technological opportunities based on patents: text extract and term analysis. Technovation 60-61, 1–14 (2017). Org / 10.1016 / J.Techovation.2017.03.001. Accessed in 2022-09-30 [10] Blouin, M., SERY, N., Cluzeau, D., Brun, J.-J., BEDECARRATS, A. Environmental Management 52 (2), 309–320 (2013). Org / 10.1007 / S00267-013-0079-8. Accessed in 2022-10-19 [11] Chand Bhatt, P., Kumar, V., Lu, T.-C., Daim, t.: Evaluation of technological convergence: Blockchain case in the IR 4.0 platform. Technology in Society 67, 101709 (2021). Org / 10.1016 / J.TECHSOC.2021. 101709. Accessed 2022-10-20 [12] Kim, TS, SOHN, SY: Deep semantic analysis approach based on machine learning for forecasting the convergence of new technologies. Technological forecast and social change 157, 120095 (2020). Org / 10.1016 / J.TECHFORE.2020.120095. Consulted 2022-10-20 [13] Li, H., An, H., Wang, Y., Huang, J., GAO, X.: Evolutionary characteristics of the network of co-targeted words of academic articles and the network of keywords of keywords: based on a two-modes affiliation network. Physica A: statistical mechanics and its applications 450, 657–669 (2016). Org / 10.1016 / d. Physa. 2016.01.017. Accessed in 2022-09-09 [14] Chen, H., Zhang, Y., Zhu, D.: Identification of technological subject changes in patent claims using the subject's modeling. In: DAIM, Tu, Chiavetta, D., Porter, Al, Saritas, O. (ed.) Anticipate future pathways through a great data analysis. Innovation, technology and knowledge management, pp. 187–209. Springer, Cham (2016). Org / 10. 1007 / 978-3-319-39056-7 11 [15] COJOCARU, S., BRAGARU, C., Ciuchi, O.: The role of language in the construction of social realities. The grateful investigation and the reconstruction of organizational ideology. Revista de Cercetare if Sociala 36, 31–43 (2012) [16] He, C., Shi, F., Tan, R.: A method of synthetic analysis of measuring technological convergence. Expert systems with Applications 209, 118262 (2022). ORG / 10.1016 / J.ESWA.2022.118262. Accessed 2022-10-21 [17] Klarin, A., Suseno, Y., Lajom, J.: Revue of the systematic literature of convergence: perspective of systems and re -evaluation of the convergence process. IEEE transactions on engineering management, 1–13 (2021). Org / 10.1109 / TEM.2021.3126055 [18] Macroberts, MH, Macroberts, BR: Citation analysis problems. Sciencetometry 36 (3), 435–444 (1996). Org / 10.1007 / BF02129604. Consulted 2022-11-23 [19] Liu, P., Xia, H.: Structure and evolution of the co-automation network in an area of interdisciplinary research. Scientometrics 103 (1), 101–134 (2015). Org / 10.1007 / S11192-014-1525-Y. Consulted 2022-11-30 [20] Lu, L., Zhou, t.: Liaison prediction in complex networks: an investigation. Physica A: Statistical mechanics and its applications 390 (6), 1150–1170 (2011). [org/10.1016/j.physa.2010.11.027. Accessed 2022-10-17 ](Org / 10.1016 / J.Physa.2010.11.027. Consulted 2022-10-17) [21] Kim, J., Kim, S., Lee, c .: Anticipation of technological convergence: prediction of links using Wikipedia hyperlinks. Technotation 79, 25–34 (2019). Org / 10.1016 / J.Techovation. 2018.06.008. Accessed 2022-10-21 [22] Breitzman, A., Thomas, p.: The model of emerging clusters: a tool to identify emerging technologies on several patent systems. Research policy 44 (1), 195-205 (2015). Org / 10.1016 / J. RESPOL.2014.06. 006. Consulted 2022-10-21 [23] Kumar, T., Vaidyanathan, S., Annanthapadmanabhan, H., Parthasarathy, S., Ravindran, b.: Hypergraph clustering by the maximization of modularity by iterative. Applied Network Science 5 (1), 1–22 (2020). Org / 10.1007 / S41109-020-00300-3. Number: 1 publisher: Springeropen. Consulted 2022-10-20 [24] Schoen, A., Villard, L., Laurens, P., Cointet, J.-P., Heimeriks, G., Alkemade, F.: The structure of the network of technological developments; Technological distance as a walk on the technological map (2012) [25] Kim, J., Lee, s.: Forecast and identification of multi-technical convergence based on patent data: the case of computer industries and BT in 2020. Sciencetometry 111 (1), 47–65 (2017). Org / 10.1007 / S11192-017-2275-4. Accessed 2022-10-21 [26] Lee, C., Kwon, O., Kim, M., Kwon, D.: Early identification of emerging technologies: an automatic learning approach using several patent indicators. Technological forecast and social change 127, 291–303 (2018). Org / 10.1016 / J.TECHFORE.2017.10.002. Accessed in 2022-10-17 [27] Kajikawa, Y., Takeda, Y.: Research structure on biomass and bio-finites: an approach based on quotation. Technological forecast and social change 75 (9), 1349–1359 (2008). Org / 10.1016 / J.TECHFORE.2008.04.007. Accessed in 2022-10-18 [28] Mitrea, C., Lee, C., Wu, Z.: A comparison between neural networks and traditional forecasting methods: a case study. International Journal of Engineering Business Management 1 (2009). Org / 10.5772 / 6777 [29] Priem, J., Piwowar, H., Orr, R.: OpenAlex: an entirely open index of works, authors, places, institutions and fully open concepts. Arxiv. Arxiv: 2205.01833 [cs] (2022). Org / 10.48550 / Arxiv. 2205.01833. Accessed in 2022-10-03 [30] Brown, BB, et al.: Delphi process: a methodology used for the elicitation of expert opinions. Rand Corporation Santa Monica, CA (1968) [31] Rousseeuw, PJ: Silhouettes: graphic help for the interpretation and validation of the analysis in clusters. Journal of Computational and Applied Mathematics 20, 53–65 (1987)Authors:
(1) Alessandro Tavazz, Cyber Defense Campus, Armasuisse Science and Technology, Building I, EPFL Innovation Park, 1015, Lausanne, Switzerland, Institute of Mathematics, EPFL, 1015, Lausanne, Switzerland and corresponding author (Author corresponding (Author corresponding (Author corresponding (Author corresponding ([email protected]));
(2) Dimitri Percia David, Cyber Defense Campus, Armasuisse Science and Technology, Building I, EPFL Innovation Park, 1015, Lausanne, Switzerland and Entrepreneurship and Management Institute, University of Applied Sciences from Western Switzerland (HES-SO Valais-Wallis), Techno-Pole 1, Le Foyer, 3960, Sierre, Switreland;
(3) Julian Jang-Jaccard, Cyber Defense Campus, Armasuisse Science and Technology, Building I, EPFL Innovation Park, 1015, Lausanne, Switzerland;
(4) Alain Mermoud, Cyber Defense Campus, Armasuis Science and Technology, Building I, EPFL Innovation Park, 1015, Lausanne, Switzerland.