Enhancing Trauma and Acute Care with AI-Driven Decision Support Systems
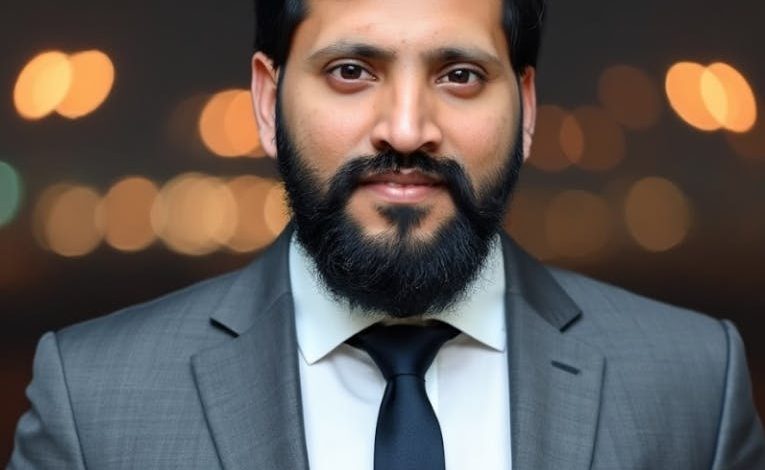
In one of his research articles published in Nanotechnology Perceptions, Tulasi Naga Subhash Polineni presented an interesting vision of the integration of generative neural networks and artificial intelligence in trauma and active care systems. This collaborative study explores how
With more than a decade of experience in Oracle Technologies, Cloud Computing and Business Integration, Polineni has history of resolution of the challenges of real world health taking advantage of its technical sense. Thanks to his research, he highlighted the potential of generative neural networks, the integration of data in real time and predictive analysis to improve clinical decision -making in trauma environments.
Emergency care via AI
Emergency services (EDS) are extremely high intensity of resources that are known to operate under enormous pressure, assistant to patients with various degrees of severity and often missing or incomplete health files. Clinicians of these units are often necessary to make decisions that change life within tight deadlines on the basis of limited information. Any ineffectiveness of sorting or delay in the diagnosis can considerably affect patient results in such environments.
Thanks to his research, Polineni recommended a fundamental change in the way in which installations structure emergency care. He mentions that clinical decision -making can be increased with data focused on data by introducing AI tools. These systems can analyze large sets of data in real time, in particular patient stories, environmental variables, imaging results and physiological data to generate probabilistic assessments of gravity and risk. By identifying critical conditions before the symptoms degenerate, AI allows emergency services to pass reactive care for proactive care.
Diagnostic and sorting procedures can also be standardized by AI, which reduces human error and subjectivity. This can be extremely beneficial in subressour or rural areas where the availability of trained specialists can be a concern. In order to reduce diagnostic errors, increase efficiency and save lives, hospitals can integrate smart tools throughout their emergency intervention workflow.
Early diagnosis and prognosis
The basic concept of Polineni's research revolves around advanced models of generative neural networks such as generative opponents (GAN) and variational autoencoders (VAE). These models are extremely effective in early diagnosis and prognostic modeling, two most acute challenges in trauma care. These models are tailor -made to overcome practical problems such as the variability in patient presentations, data shortage and the lack of expert labeling consistent in clinical data.
Generative opponent networks (GAN) are able to generate synthetic data from diagnostic mirror patients or real world imaging, which provides training data sets for under-represented or rare conditions. On the other hand, variational auto -enteencoders (VAE) can effectively compress and reconstruct medical images. This improves the detection of characteristics and models that could escape the human eye.
It is possible to train these models to identify the subtle indicators of conditions such as sepsis, internal bleeding, intracranial hemorrhage and even a complex multi-organization failure in times. These tools can allow doctors to make decisions reflecting the immediate and long -term implications of the trauma.
Real -time decision -making aid systems
The concept of decision -making systems has been used in the health care sector for many years. However, Polineni says that the integration of
Adaptability is one of the key compatibility of these systems. Whether it is deployed in a distant field hospital or an animated metropolitan trauma center, the DSS in real time can evolve according to the requirement. They help with the allocation of resources, prioritize patients during an increase and even coordinate the transfers of care between the facilities.
AI to improve sorting and emergency interventions
As this is the fundamental step in emergency care, any delay or bad classification at the sorting stage can cause potentially fatal consequences. In his study, Polineni has discussed improved AI sorting models which are not only capable of accelerating the sorting of patients, but also the improvement of precision in consideration in subtle indicators that human indicators tend to fail.
Using large data sets to train generative models, these systems can detect critical conditions such as traumatic brain lesions, internal bleeding or the first signs of sepsis. By helping to follow the disposition of patients, AI ensuring the continuity of care of prehospital environments to specialized treatment facilities.
Field applications
Polineni illustrated the impact of DSS led by AI through a series of real world use cases.
- Pediatric trauma sorting: By forming AI tools on data sets specific to pediatrics, clinicians can be helped to identify the models of atypical vital signs. This can help detect serious injuries such as concussion or internal bleeding in time.
- Emergency radiology interpretation: AI algorithms formed on thousands of annotated images can precisely identify anomalies in MRI or CT analyzes. By highlighting the alleged areas of concern, these tools allow radiologists to reduce interpretation time during peak hours and prioritize critical cases.
- Pre -hospital trauma response: Equipped with AI assisted mobile devices, emergency stakeholders can enter the basic patient data on site. Based on this data, the system generates a gravity index and recommends the most appropriate installation.
- Evaluation of the severity of burns and wounds: Based on historical results, IA -improved image analysis can detect the risk of infection, classify burning depths and recommend treatment protocols.
Final reflections
Tulasi Naga Subhash Polineni's research has created a full plan that can define the future of trauma and acute care by drawing a means of AI decision -making systems.
“Our goal is not only to improve the accuracy of the diagnosis, but to fundamentally rethink how trauma and acute care can be provided, smarter and more personalized,” concludes Tulasi Naga Subhash Polineni. “AI -focused decision -making systems have the potential to fill in intensive care gaps, reduce diagnostic delays and, ultimately, save lives in emergencies sensitive to time.