What Is Text Sanitization? Definitions, Privacy Laws, and NLP Approaches
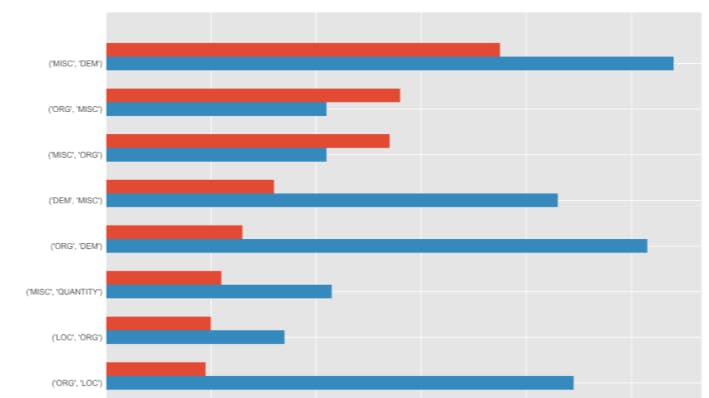
Authors:
(1) Anthi Papadopoulou, Language Technology Group, University of Oslo, Gaustadalleen 23b, 0373 Oslo, Norway and corresponding author ([email protected]));
(2) Pierre Lison, Norwegian Computing Center, Gaustadalleen 23a, 0373 Oslo, Norway;
(3) Mark Anderson, Norwegian Computing Center, Gaustadalleen 23a, 0373 Oslo, Norway;
(4) Lilja Øvrelid, Language Technology Group, University of Oslo, Gaustadalleen 23b, 0373 Oslo, Norway;
(5) Ildiko Pilan, Language Technology Group, University of Oslo, Gaustadalleen 23B, 0373 Oslo, Norway.
Ties
Summary and 1 Introduction
2 context
2.1 Definitions
2.2 NLP approaches
2.3 Data edition preserving confidentiality
2.4 Differential confidentiality
3 data sets and 3.1 Benchmark Anonymization of the text (tab)
3.2 Wikipedia biographies
4 Recognize private life entities
4.1 Wikidata properties
4.2 Silver corpus and fine model adjustment
4.3 Evaluation
4.4 Disagreement labeled
4.5 Various semantic type
5 risk of confidentiality indicators
5.1 LLM probabilities
5.2 SPAN classification
5.3 Disturbances
5.4 Sequences labeling and 5.5 Web search
6 Analysis of confidentiality risk indicators and 6.1 evaluation metrics
6.2 Experimental results and 6.3 discussion
6.4 Combination of risk indicators
7 conclusions and future work
Statements
References
Annexes
A. Wikidata human properties
B. Training parameters for recognition of entities
C. Label Agreement
D. LLM probabilities: Basic models
E. Size and performance of the training
F. Disturbance thresholds
2.1 Definitions
The right to privacy is a fundamental human right, as evidenced by its inclusion in the Universal Declaration of Human Rights and the European Convention on Human Rights. In the digital sphere, data confidentiality is applied through several national and international regulations, such as the General Data Protection Regulations (GDPR) in Europe, California Consumer Privacy Act (CCPA) in the United States or the Chinese Information Protection Act (PIPL). Although these regulations differ both in scope and interpretation, their common principle is that individuals must keep control of their own data. In particular, the processing of personal data must have legal ground and cannot be shared to third parties without the explicit and informed consent of the data (s) to data.
An alternative strategy consists of anonymize The data to ensure that the data is no longer personal, and therefore outside the extent of the confidentiality regulations. Anonymization, according to the GDPR, refers to the complete and irrevocable deletion of all the information which can directly or indirectly lead to a re -identification. However, as Weitzenboeck et al shows. (2022), data transformation to make them completely anonymous is almost impossible to perform in practice for unstructured data such as text, unless the content of the text is radically modified or that the original source of the document is deleted.
Although complete anonymization is difficult to reach, text disinfection is a crucial tool to adhere to the general requirement of Data minimization which is devoted to the GDPR and most confidentiality regulations (Goldsteen et al., 2021). The principle of data minimization stipulates that it is not necessary to collect and keep personal data strictly necessary to achieve a given objective.
The process of publishing text documents to hide the identity of a person has somewhat confusing terminology (Lison et al., 2021; Pil´an et al., 2022). The GDPR uses the term pseudonymization to designate a data transformation process to hide at least certain personal identifiers, but in a way that does not equivalent to finishing anonymization. The term identification is also common (Chevrier et al., 2019; Johnson et al., 2020), in particular for work on patient medical records. Disidentification approaches are generally limited to the recognition of predefined entities, such as the categories of HIPAA (2004). On the other hand, we define text disinfection Like the detection and masking process of any type of personal information in a text document which can lead to the identification of the individual whose identity we want to protect.
Text disinfection is a subject of investigation in several areas of research, in particular in the treatment of natural language (NLP) and in the publication of data preserving confidentiality (PPDP). Text rewriting approaches based on differential confidentiality have also been proposed. We examine these approaches below.
2.2 NLP approaches
PNL's approaches to text disinfection were mainly focused on sequence labeling approaches, inspired by the major work body on the recognition of named entities. Such approaches aim at the detection of text spans containing personal identifiers (Chiu and Nichols, 2016; Lample et al., 2016). Most research work in this area to date have focused on the medical field, where the 1996 law on health insurance and responsibility (HIPAA, 2004) offers concrete rules that allow the normalization of this task. HIPAA defines a set of types of protected health information data (PHI) which include direct identifiers (such as names or social security numbers) as well as demographic attributes specific to the domain, including treatments received and health problems. A wide variety of NLP methods have been developed for this task, including rules based on rules, based on automatic and hybrid learning (Sweeney, 1996; Neamatullah et al., 2008; Yang and Garibaldi, 2015; Yogarajan et al., 2018). The networks of recurring neurons based on the characters (Dernoncourt et al., 2017; Liu et al., 2017) and the architectures of transformers have also been studied for this purpose (Johnson et al., 2020). A recent initiative focused on the replacement of sensitive information is incognitus (Ribeiro et al., 2023), a tool for the identification of the clinical note. The system allows you to restart documents with a NER method or with an approach based on integration substituting all tokens with a semantically approach. Recent recent models from the GPT family have also been explored. Liu et al. (2023) proposed the DEID-GPT for the masking of Phi categories and showed that with learning in the zero context incorporating explicit HIPAA requirements in the invites, GPT-4 has surpassed models of refined transformators on the same annotated medical texts.
Text disinfection outside the medical field includes approaches such as Juezhernandez et al. (2023), which offers Agora, a document of identification of document combined with geoparsing (automatic extraction of the location from text) using LSTMS and CRFs and trained on Spanish law enforcement. The authors focus on providing a complete pipeline and location information, while demographic attributes are not part of the information to be identified. Yermilov et al. (2023) compared three systems for the detection and pseudonymization of the PII: (1) a nerge based on a nerve on Wikidata; (2) a sequence sequence model in a single step formed on a parallel corpus; and (3) a large language model where the named entities are first detected using a 1 stroke invite to GPT-3, then pseudonymized with 1 stroke prompts using Chatgpt (GPT-3.5). The authors note that the NER -based approach is the best to preserve confidentiality while the LLMs preserve the best utility for a text classification and summary tasks. Finally, Papadopoulou et al. (2022) present an approach to text disinfection, detecting personal information to estimate the risk of confidentiality thanks to the use of the probabilities of the language model, web requests and a classifier formed on manually labeled data. The current document is based on this work.