How Text Span Analysis Enhances Data Privacy
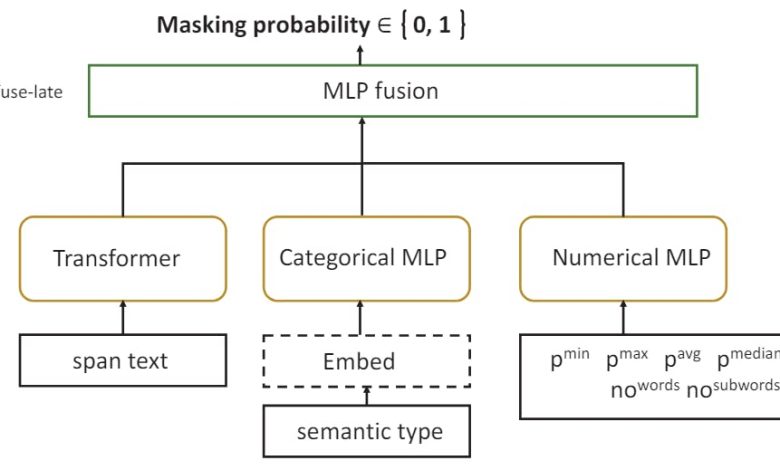
Those with -set:
.[email protected]);
(2) Pierre Lison, Norwegian Computing Center, Gaustalleen 23A, 0373 Oslo, Norway;
(3) Mark Anderson, Norwegian Computing Center, Gaustalleen 23A, 0373 Oslo, Norway;
.
.
Link
Abstract and 1 Introduction
2 background
2.1 definitions
2.2 approach to NLP
2.3 Publishing privacy data
2.4 Differences in Difference -It
3 datasets and 3.1 text anonymization benchmark (tab)
3.2 Wikipedia biography
4 Privacy-Oriented Entity Recognition
4.1 Wikidata Characteristics
4.2 Silver Corpus and Model Fine-Tuning
4.3 Analysis
4.4 Label Disagreement
4.5 misc semanty type
5 Privacy Danger indicators
5.1 LLM probabilities
5.2 SPAN CLASS
5.3 perturbations
5.4 Sequence Labeling and 5.5 Web Search
6 Analysis of Privacy Danger indicators and 6.1 Evaluation metrics
6.2 Results of Experimental and 6.3 Discussion
6.4 combination of risk indicators
7 conclusions and work in the future
Expression
References
Appendices
A. Person owners from Wikidata
B. Parameters of Entity Recognition Parameters
C. Label Agreement
D. LLM probabilities: Base models
E. Size of training and performance
F. Perturbation thresholds
5.2 SPAN CLASS
The strategy in the previous section has the benefit of being relatively general, as it only considers the combined -associated LLM probabilities and predicted PII type. However, the content of the text of the span itself is not considered. We now present an indicator that includes this text information to predict whether a length of text generates a high risk of privacy, and therefore should be a mask.
Concretely, we enriched the classifier from the previous section by adding the features of a text vector representation of the text that came from a large language model. The classification thus depends on a combination of the feature numbers (the combined -coincidence of LLM discussed above, to which we added the number of words and subwords of the span), a classification feature (a PII type type), and a vector representation of the span span. Crucially, the transformer -based language model that makes this vector representation work well with this prophecy work.
The resulting architecture of the model is described in Fig. Concretely, model training is done using the Autogluon's multimodal predictor (Shi et al., 2021), making it easier to include a wide range of features, including textual content processed in a neural language model. We use the Electra's discriminator model here because of the efficient performance of the Sentence (Clark et al., 2020; Shi et al., 2021). The classification and number features are processed by a standard MLP. After each model is trained separately, their output is pool (concatenation) of an MLP model using a fuse-late approach near the output layer. Table 4 provides some examples of input.
For our experiments, we re -adapt to the classifier using datasets of annotated annotated collection of Wikipedia's biography and the Tab Corpus.
5.3 perturbations
The two previous risk indicators use features obtained from the length of the text to emulate masking decisions from human anotators. They do not, however, will be able to develop how much information a PII SPAN can contribute to re-identification. They also consider each PII span in separation, thus ignoring the fact that quasi-identifiers develop a privacy risk precisely because of their marriage with one another. We now turn to an indicator of privacy risk aimed at meeting those two challenges.
This method is inspired by salience methods (Li et al., 2016; Ding et al., 2019) used to explain and interpret the outputs of large language models. One of this methods is that one changes the model's input by either change or removal, and observes how this change affects the prophecies (Kindermans et al., 2019; Li et al., 2017; Serrano and Smith, 2019). In our case, we Perturb The input by changing one or more PIIs covers and studies the consequences of this change in the possibility of the language model made for another PII span in the text.
Consider the following text:
To check which mask covers, we use a large roberta model (Liu et al., 2019) and all possible PII combinations in the text in a perturbation -based setting. We start by calculating the possibility of a target span with the remaining context available in the text. We then mask each other PII span in the document and recover the probability of the target span to determine how the PII span absence affects the possibility of the target span.
To obtain smart estimates, we must mask all spans that define the same creature while calculating the possibilities. For example 9, Michael Linder and Linder Refer to the same creature. We use in our experiments the co-reference links annotated in Tab Corpus and the collection of Wikipedia biographies.