Improving Privacy Risk Detection with Sequence Taging and Web Search
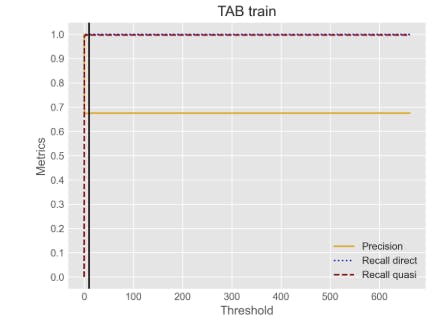
Authors:
(1) Anthi Papadopoulou, Language Technology Group, Oslo University, Gaudadalleen 23b, 0373 Oslo, Norway and corresponding author ([email protected]);
(2) Pierre Lison, Norwegian Calculation Center, Gaudadalleen 23a, 0373 Oslo, Norway;
(3) Mark Anderson, Norwegian computing center, Gaudadalleen 23a, 0373 Oslo, Norway;
(4) Lilja Øvrelid, Language Technology Group, Oslo University, Gaudadalleen 23b, 0373 Oslo, Norway;
(5) Ildiko Pilan, Language Technology Group, Oslo University, Gaudadalleen 23b, 0373 Oslo, Norway.
To links
Summary and 1 Introduction
2
2.1 Definitions
2.2 NLP approaches
2.3 Disclosure of Privacy Maintain Data
2.4 Difference Privacy
3 Data sets and 3.1 Text anonymized benchmark (tab)
3.2 Wikipedia biographies
4 Privacy -oriented item recognition
4.1 Wikida properties
4.2 Silver Corpus and Model Fine Tuning
4.3 Evaluation
4.4 Tag Disagreements
4.5 MISC Semantic Type
5 Privacy risk indicators
5.1 LLM probabilities
5.2 Classification
5.3 PERTURBATIONS
5.4 Song Signing and 5.5 Web Search
6 Analysis of Privacy Risk and 6.1 Assessment Metrics Analysis
6.2 Experimental results and 6.3 discussion
6.4 A combination of risk indicators
7 conclusions and future work
Declarations
References
Extras
A. Wikida human qualities
B. The training parameters of the entity of the unit
C. Tag contract
D. LLM probabilities: basic models
E. The size and performance of training
F. Perturbation thresholds
5.4 Tagging
Another approach to assessing the risk of re -identification of experts on masking decisions is an assessment of the sequence labeling model. Compared to previous methods, this method most depends on the availability of intra -domain, marked training data.
For this approach, we specify the code -type language model for the classification of the mark, each character is either assigned to the mask or there is no mask. For Wikipedia's biographies, we rely on the Roberta model (Liu et al., 2019), while the Longformer Model (Beltagy et al., 2020), given the length of lawsuits, as proposed in Pil´an and others. (2022). Due to the discrepancies of hand -marked distances or, the detected privacy -oriented entrepreneur, and due to the funds created by a subtle voice model, we act under two possible settings:
!
• Partial match: We think the spa is high at risk if the Longformer/Roberta model marks at least one mark as a mask.
5.5 Web Search
We used Google API in a given document for each target audience and unique text plans that appear in this document[7]To. Google API gives 10 results per page. We limit the test with the top 20 results (ie the first two pages of the web tip). To avoid too many API calls, we also restrict the search for individual text extensions, although in principle, the same approach can be extended to the combinations of peak lengths.
We also used the total number of hits reported by Google Search for each Pi Span query. It is assumed that if the search gives more answers, one of these answers is more likely to contain information on the target. However, general search queries are likely to return many answers. Therefore, we considered the use of the upper and lower bounds of the total numbers. These thresholds were experimentally set to maximize the Token level F1 tab. This resulted in a lower limit of 100 hits and the upper limit. This method is restricted by the potential unreliable nature of the total reactions presented by web search engines, as shown by S´anchez et al. (2018).
[7] Web searches date back to July 2023 to September 2023.